Thursday and Friday February 19-20, 2009
Autodesk Technologist with Information about Stormwater Management Model (SWMM) for watershed water quality, hydrology and hydraulics modelers (Note this blog is not associated with the EPA). You will find Blog Posts on the Subjects of SWMM5, ICM SWMM, ICM InfoWorks, InfoSWMM and InfoSewer.
Saturday, January 17, 2009
International Conference on Stormwater and Urban Water Systems Modeling
Thursday and Friday February 19-20, 2009
Saturday, January 3, 2009
📊 SWMM 5 Complexity Index 📊
📊 SWMM 5 Complexity Index 📊
The SWMM 5 Complexity Index offers a way to measure a model's intricacy against a benchmark: the first Extran example in Extran 3, now referred to as network #1 in this broader SWMM 5 context. The foundational network showcases 22 objects and runs simulations over 8 hours. 🕗 Notably, it took 5 minutes to process this network on an IBM AT back in 1988. 🖥️⏳
The core aim of this complexity index? To provide a comparative tool for contemporary models. 📈🔄 The complexity formula evaluates the object count in the new model versus the baseline, while also accounting for any extensions in simulation time. 🔄🔍📏
📊 Complexity Index Breakdown 📊
The complexity index consolidates the count of various elements: raingages, subcatchments, junctions, outfalls, dividers, storages, conduits, pumps, orifices, weirs, outlets, control curves, and many more, right up to snowpack objects. 🌧️🌍🚰🔀🌊
For a more nuanced understanding, this index is then amplified by tallying pollutants across various elements like subcatchments, junctions, or weirs. Additionally, the multiplication of the number of land uses by the count of subcatchment objects is considered. 🧪🔄🌳🏘️
To gauge its relative complexity, this index is juxtaposed against network #1. This involves dividing the freshly computed complexity index by the foundational 22 objects and contrasting the new network's duration against the 8-hour benchmark of the base network. 🕗📏 The exemplified network flaunts a complexity rating of 5.2 and, impressively, executes in under a second on an Intel Dual Core Processor. 🖥️⚡
🔍 Understanding the Complexity Index
The complexity index is a comprehensive metric that sums up various components of a given hydrologic model. Specifically, it aggregates:
Rain gauges, subcatchments, junctions, outfalls, dividers, storages, conduits, pumps, orifices, weirs, outlets, and several curve types (control, diversion, pump, rating, shape, storage, tidal), as well as time series, patterns, transects, hydrographs, aquifers, controls, climate objects, and snowpacks. 🌦️🌍🚰
The index is then adjusted by taking into account the number of pollutants for multiple components like subcatchments, junctions, outfalls, and so forth. 🧪
Additionally, it factors in the number of land uses multiplied by the count of subcatchment objects. 🌲🏙️
📏 Comparing the Complexity Index:
To gauge the relative complexity of a network:
- The computed complexity index is divided by a baseline value of 22 objects. 📊
- The duration of the new network is normalized against an 8-hour duration of a reference network. 🕗
For example, a showcased network had a complexity index of 5.2 and executed in under a second on an Intel Dual Core Processor. 💨🖥️
📂 Complexity Indices from Sample Models:
Using the EPA SWMM 5 QA/QC suite of files, the complexity indices for different models in the DATA.ZIP file are:
- USER4.INP: 88.5 📈
- USER1.INP: 7.4 📉
- USER2.INP: 55 📊
- USER3.INP: 20.1 📉
- USER5.INP: 18.5 📉
In essence, the complexity index provides a quantitative measure of a hydrologic model's intricacy, enabling modelers to benchmark and optimize performance efficiently. 👩💼🔧📊
Friday, December 26, 2008
SWMM 5 Pond Infiltration
Thursday, December 25, 2008
SWMM 5 Variable Time Step

Sunday, December 21, 2008
SWMM5 Normal Flow
If the link gets to the Check Normal Flow routines then it uses the following logic:
- If the Slope or Both option is used or either the upstream node or the downstream node of the link is an outfall AND Y1 is less than Y2 then the minimum of Q from the dynamic wave equation or Q from the Normal Flow equation is used as the current iteration flow in link, or
- If the Froude or Both option AND either the upstream Froude Number or the downstream Froude number is greater than 1 then the minimum of Q from the dynamic wave equation or Q from the Normal Flow equation is used as the current iteration flow in link. This condition is never used if either of the connecting nodes of the link are outfalls.
How does this work in the actual flow that SWMM 5 estimates for a link? Consider this example in which the link flow in blue is plotted with the Qnormal flow in red and the Q dynamic wave equation flow in purple:
Qnormal is
Qnormal is only calculated when the link is not full so in the plot a Qnormal of 0 means that the pipe was full. At other times the flow in the link was equal to Qnormal as the minimum of the dynamic equation flow or the Qnormal flow is used at each iteration in the solution process. The flow is normally bounded by the Qnormal flow in SWMM 5.0.013. Your choice of the options Slope, Froude andBoth really only impact the conditions under which this comparison is true. If you use Froude or Both then Supercritical flow at either end of the link will trigger this comparison will be the dynamic wave equation flow and the Froude number at each end of the link.
Smaller Storms Drop Larger Overall Rainfall In Hurricane Season
ScienceDaily (Dec. 11, 2007) — Researchers have found that when residents of the U.S. southeastern states look skyward for rain to alleviate a long-term drought, they should be hoping for a tropical storm over a hurricane for more reasons than one. According to a new study using NASA satellite data, smaller tropical storms do more to alleviate droughts than hurricanes do over the course of a season by bringing greater cumulative rainfall.
A new study that provides insight into what kind of storms are best at tackling drought in the southeastern United States. The study focuses on a decade of first-ever daily rainfall measurements by a NASA satellite carrying a weather radar in space. The study's authors believe the same insights can be applied by meteorologists and public officials to other regions where daily satellite rainfall data and storm tracking data are available.
In the wake of Hurricane Katrina, meteorologist Marshall Shepherd, an associate professor of geography and atmospheric sciences at the University of Georgia, Athens, and colleagues delved into the ongoing debate about whether global warming is leading to an increase in rainfall intensity. The researchers wanted to determine how much rainfall each type of cyclone, from tropical depressions to category five hurricanes, contributes to overall rainfall. They focused the study on the Southeast in the hope that results could be harnessed to improve drought relief information for the region. Their findings were published today in the American Geophysical Union's Geophysical Research Letters.
"As much of the Southeast experiences record drought, our findings indicate that weak tropical systems could significantly contribute to rainfall totals that can bring relief to the region," said Shepherd, lead author of the NASA-funded study. "These types of storms are significant rain producers. The larger hurricanes aren't frequent enough to produce most of the actual rain during the season and therefore are not the primary storm type that relieves drought in the region."
Shepherd created a new measurement method as an efficient way to get a real sense for how much rainfall each type of storm contributes in a given year around the coastal regions of the southeastern U.S. To do so, he had to distinguish an average rainfall day from an extreme rainfall day. Though data from NASA's Tropical Rainfall Measuring Mission (TRMM) satellite could offer daily rainfall amounts, the data could not be used to set apart whether rainfall was average or extreme for any given day.
Shepherd and his team modeled their metric on the "cooling degree day" that energy companies use to relate daily temperature to energy needs for air conditioning. A cooling degree day is found by subtracting 65 degrees from the average daily temperature. Values larger than zero give some indication whether a day was abnormally warm. Shepherd used daily rainfall data from TRMM to determine 28.9 as the base value of average daily rainfall at one of the world's wettest locations, Maui's Mount Wailea in Hawaii.
In the same way as the cooling degree day, the "millimeter day" metric is calculated by subtracting 28.9 millimeters from the average daily rainfall in each of four ocean basins along coastal areas scattered across the south near Houston and New Orleans, east of Miami and south of North Carolina. Values greater than zero indicate a so-called "wet millimeter day" of extreme rainfall.
Using daily rainfall data from the TRMM satellite from 1998-2006, Shepherd's team compared the amount of rain that fell in the basins on extreme rainfall days with the location of tropical storms from the National Hurricane Center's storm tracking database to determine how many extreme rainfall days were associated with a particular type of tropical storm.
The team found that the most extreme rainfall days occurred in September and October, two of the busiest months of the Atlantic hurricane season. They also found that though major hurricanes produced the heaviest rainfall on any given day, the smaller tropical storms and depressions collectively produced the most rainfall over the entire season. Over half of the rainfall during the hurricane season attributed to cyclones of any type came from weaker tropical depressions and storms, compared to 27 percent from category 3-5 hurricanes.
TRMM has transformed the way researchers like Shepherd measure rainfall by providing day-to-day information that did not exist before the satellite's 1997 launch. "Though we've had monthly rainfall data available since 1979 from other sources, it's the daily rainfall data that allows us to see that tropical storm days contributed most significantly to cumulative rainfall for the season due to how frequently that kind of storm occurs," said Shepherd.
"It's important in the future to build a longer record of daily rainfall to establish, with better confidence, whether trends are occurring," said Shepherd. "This study sets the stage for us to understand how much rainfall weak and strong tropical cyclones contribute annually and whether this contribution is trending upward in response to global warming-fueled growth in tropical cyclones."
Shepherd believes advances that will improve study of cyclones and rainfall are "just around the corner" with NASA's Global Precipitation Measurement satellite, scheduled for launch in 2013. An extension of TRMM's capabilities, it will measure precipitation at higher latitudes, the actual size of snow and rain particles, and distinguish between rain and snow.
Adapted from materials provided by NASA/Goddard Space Flight Center, via EurekAlert!, a service of AAAS.
Email or share this story:
Need to cite this story in your essay, paper, or report? Use one of the following formats:
APA
MLA
NASA/Goddard Space Flight Center (2007, December 11). Smaller Storms Drop Larger Overall Rainfall In Hurricane Season. ScienceDaily. Retrieved November 27, 2008, from http://www.sciencedaily.com /releases/2007/12/071210104022.htm
Sunday, November 30, 2008
How to Make an InfoSWMM model from the DBF Files
Monday, November 24, 2008
Force Main Transition in SWMM 5
1. If the force main is full then the program will use either Hazen-Willams or Darcy-Weisbach to calculate the friction loss (term dq1),
2. If the force main is NOT full then the program will use Manning's Equation.
// --- compute terms of momentum eqn.:
// --- 1. friction slope term
if ( xsect->type == FORCE_MAIN && isFull )
dq1 = dt * forcemain_getFricSlope(j, fabs(v), rMid);
else dq1 = dt * Conduit[k].roughFactor / pow(rWtd, 1.33333) * fabs(v);
double forcemain_getFricSlope(int j, double v, double hrad)
//
// Input: j = link index
// v = flow velocity (ft/sec)
// hrad = hydraulic radius (ft)
// Output: returns a force main pipe's friction slope
// Purpose: computes the headloss per unit length used in dynamic wave
// flow routing for a pressurized force main using either the
// Hazen-Williams or Darcy-Weisbach flow equations.
// Note: the pipe's roughness factor was saved in xsect.sBot in
// conduit_validate() in LINK.C.
//
{
double re, f;
TXsect xsect = Link[j].xsect;
switch ( ForceMainEqn )
{
case H_W:
return xsect.sBot * pow(v, 0.852) / pow(hrad, 1.1667); //(5.0.012 - LR)
case D_W:
re = forcemain_getReynolds(v, hrad);
f = forcemain_getFricFactor(xsect.rBot, hrad, re);
return f * xsect.sBot * v / hrad;
}
return 0.0;
}
Saturday, November 22, 2008
Friday, October 10, 2008
SCS Rainfall Distributions and Design Storms
Saturday, September 20, 2008
H2OMAP and InfoSWMM Sediment Transport Modeling
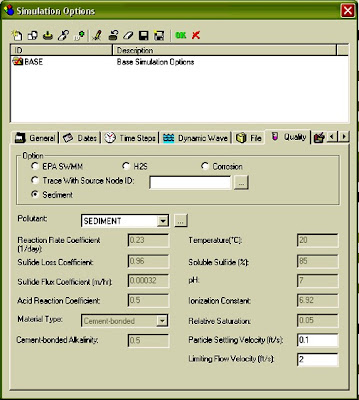
Modified Basket Handle Cross Section Warnings
Saturday, September 13, 2008
Wave Of Sewage Flows Toward Tampa Bay
Wave Of Sewage Flows Toward Bay
Sunday, September 7, 2008
SWMM 5 View Variables
SWMM 5 View Variables
There are four types of graphical variables in SWMM 5: (1) Subcatchements, (2) System, (3) Nodes and (4) Links. The SWMM 5 Hydrology binary graphics file consists of 21 view variables for each subcatcment simulation in SWMM 5. The variables are:
Subcatchment Variables | Description |
SUBCATCH_RAINFALL | rainfall intensity |
SUBCATCH_SNOWFALL | snowfall intensity |
SUBCATCH_RUNOFF | total runoff flow rate |
SUBCATCH_RUNOFF_IMPZero | runoff flow rate from zero imp area feb 2007 |
SUBCATCH_RUNOFF_IMP | runoff flow rate from imp area feb 2007 |
SUBCATCH_RUNOFF_Pervious | runoff flow rate from pervious area feb 2007 |
SUBCATCH_LOSSES | total losses (infil) |
SUBCATCH_EVAP | watershed evaporation loss |
SUBCATCH_DEPTH | watershed depth |
SUBCATCH_GW_FLOW | groundwater flow rate to node |
SUBCATCH_GW_FLOW_A1 | groundwater flow rate to node |
SUBCATCH_GW_FLOW_A2 | groundwater flow rate to node |
SUBCATCH_GW_FLOW_A3 | groundwater flow rate to node |
SUBCATCH_GW_ELEV | elevation of saturated gw table |
SUBCATCH_GW_THETA | soil moisture |
SUBCATCH_GW_PERCOLATION | aquifer deep percolation |
SUBCATCH_SNOWMELT | watershed snow melt |
SUBCATCH_SNOWDEPTH | watershed snow depth |
SUBCATCH_FREEWATER | watershed snow depth |
SUBCATCH_COLD | watershed cold content |
SUBCATCH_SNOWAREA | watershed snow coverage |
SUBCATCH_UL | soil thickness |
SUBCATCH_FTOT | infiltration during an event |
SUBCATCH_FU | current value of F |
SUBCATCH_FUMAX | maximum value of F |
SUBCATCH_MOISTURE | current soil mositure (less than porosity) |
SUBCATCH_IMD | current IMD (Porisity - Moisture) |
SUBCATCH_IMDbyEvent | IMD at the beginning of an event |
SUBCATCH_SAT | Flag for saturation (1 is saturated) |
SUBCATCH_INFIL_TIME | GA infiltration time |
SUBCATCH_WLMAX | current infiltration RATE |
SUBCATCH_NETPRECIP | rainfall intensity |
SUBCATCH_BUILDUP | pollutant buildup concentration |
SUBCATCH_WASHOFF | pollutant washoff concentration |
System Variables | Description |
SYS_TEMPERATURE | air temperature |
SYS_WINDSPEED | wind speed |
SYS_RAINFALL | rainfall intensity |
SYS_SNOWFALL | snow depth |
SYS_RUNOFF | runoff flow |
SYS_LOSSES | evap + infil |
SYS_EVAP | evap |
SYS_DWFLOW | dry weather inflow |
SYS_GWFLOW | ground water inflow |
SYS_IIFLOW | RDII inflow |
SYS_EXFLOW | external inflow |
SYS_INFLOW | total lateral inflow |
SYS_FLOODING | flooding outflow |
SYS_OUTFLOW | outfall outflow |
SYS_STORAGE | storage volume |
SYS_CE | continuity error for the basin |
SYS_ITERATIONS | average iterations over the basin |
SYS_SNOWDEPTH | snow depth |
SYS_COLD | cold storage for the basin |
SYS_SNOWMELT | snowmelt for the basin |
SYS_RAINMELT | rainmelt for the basin |
SYS_TS | time steps during the simulation |
SYS_DWFLoad | total K3 line DWF load |
SYS_WWFLoad | total K3 line WWF load |
SYS_WWFLoadExtra | agency extra WWF Load |
The SWMM 5 Node graphics binary file consists of 20 variables on one line for each junction/storage/outfall/divider simulated in SWMM 5. The variables are:
Node Variables | Description |
NODE_DEPTH | water depth above invert |
NODE_HEAD | hydraulic head |
NODE_VOLUME | volume stored & ponded |
NODE_LATFLOW | lateral inflow rate |
NODE_IIFLOW | total rdii inflow rate |
NODE_UH1 | total rdii inflow rate from UH 1 |
NODE_UH2 | total rdii inflow rate from UH 2 |
NODE_UH3 | total rdii inflow rate from UH 3 |
NODE_DWFFLOW | total DWF inflow rate |
NODE_INFLOW | total inflow rate |
NODE_OUTFLOW | total outflow rate |
NODE_OVERFLOW | overflow rate |
NODE_CE | node ce |
NODE_AREA | node surface area |
NODE_DQDH | node surcharge dqdh |
NODE_DENOM | node surcharge dqdh |
NODE_ITERATIONS | node iterations to this time step |
NODE_TIMESTEP | node iterations to this time step |
NODE_CONVERGENCE | node iterations to this time step |
NODE_QUAL | concentration of each pollutant |
Link Variables
LinkedIn algorithm prioritizes content based on several factors for SWMM5 Enablement
The LinkedIn algorithm prioritizes content based on several factors: 1. Initial Engagement (First Hour) Your post is shown to a small ...
-
@Innovyze User forum where you can ask questions about our Water and Wastewater Products http://t.co/dwgCOo3fSP pic.twitter.com/R0QKG2dv...
-
Soffit Level ( pipe technology ) The top point of the inside open section of a pipe or box conduit. The soffit is the ...
-
Engine Error Number Description ERROR 101: memory allocation error. ...